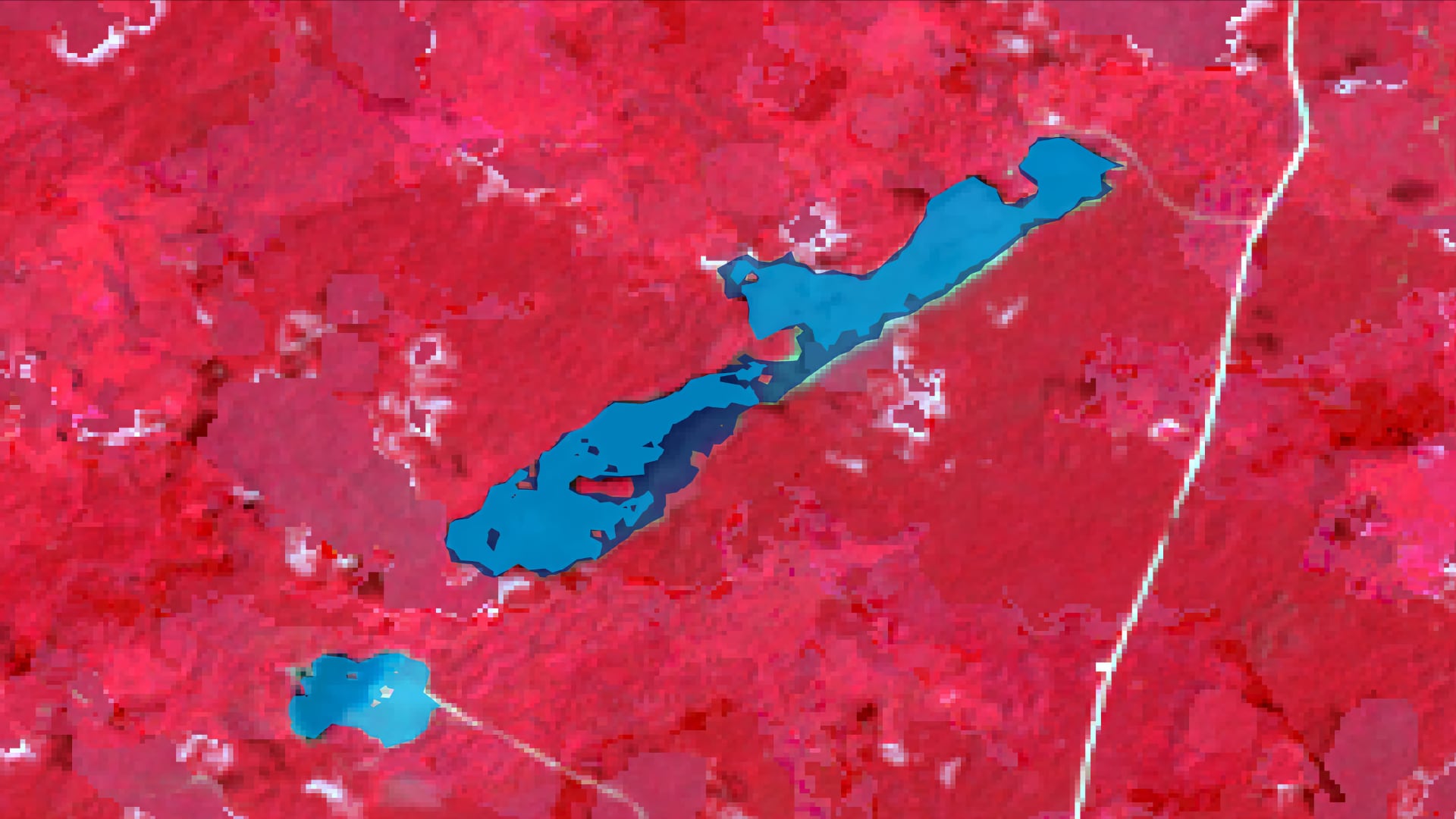
As climate change increases the severity and frequency of extreme weather events in the tropics, it is vital for the safety of local communities and the health of ecosystems to monitor seasonal inundation. Forested inundation affects the ability of forested wetlands to provide ecosystem services, such as flood mitigation, water filtration, carbon storage, and erosion mitigation. While ground-based monitoring has traditionally been used to map inundation extent, those methods are costly and time-intensive. The NASA DEVELOP team focused on seasonal inundation throughout 2008 in the Maya Forest, when changes in inundation were drastic. To monitor seasonal inundation, our team used in situ field data and Earth observations from Landsat 7 Enhanced Thematic Mapper (ETM+), Advanced Land Observing Satellite (ALOS) Phased Array type L-band Synthetic Aperture Radar (PALSAR) 1, Shuttle Radar Topography Mission (SRTM), and products from the Ice, Cloud, and Land Elevation Satellite (ICESat). The team applied a Random Forest algorithm to Landsat 7 imagery, generating an object-level land cover classification with an overall accuracy of 72.1% and forest class with 100% recall and 78% precision. The team applied L-band backscatter thresholds from existing literature to forest-masked ALOS imagery and refined the thresholds in an iterative process using field data and hydrology models to delineate seasonal inundation extent. These publicly available data products help end users from Belize’s Land Information Center (LIC) and Forest Department, Guatemala’s Center for Monitoring and Evaluation (CEMEC), and Mexico’s El Colegio de la Frontera Sur (ECOSUR) to inform land management and protect community infrastructure.