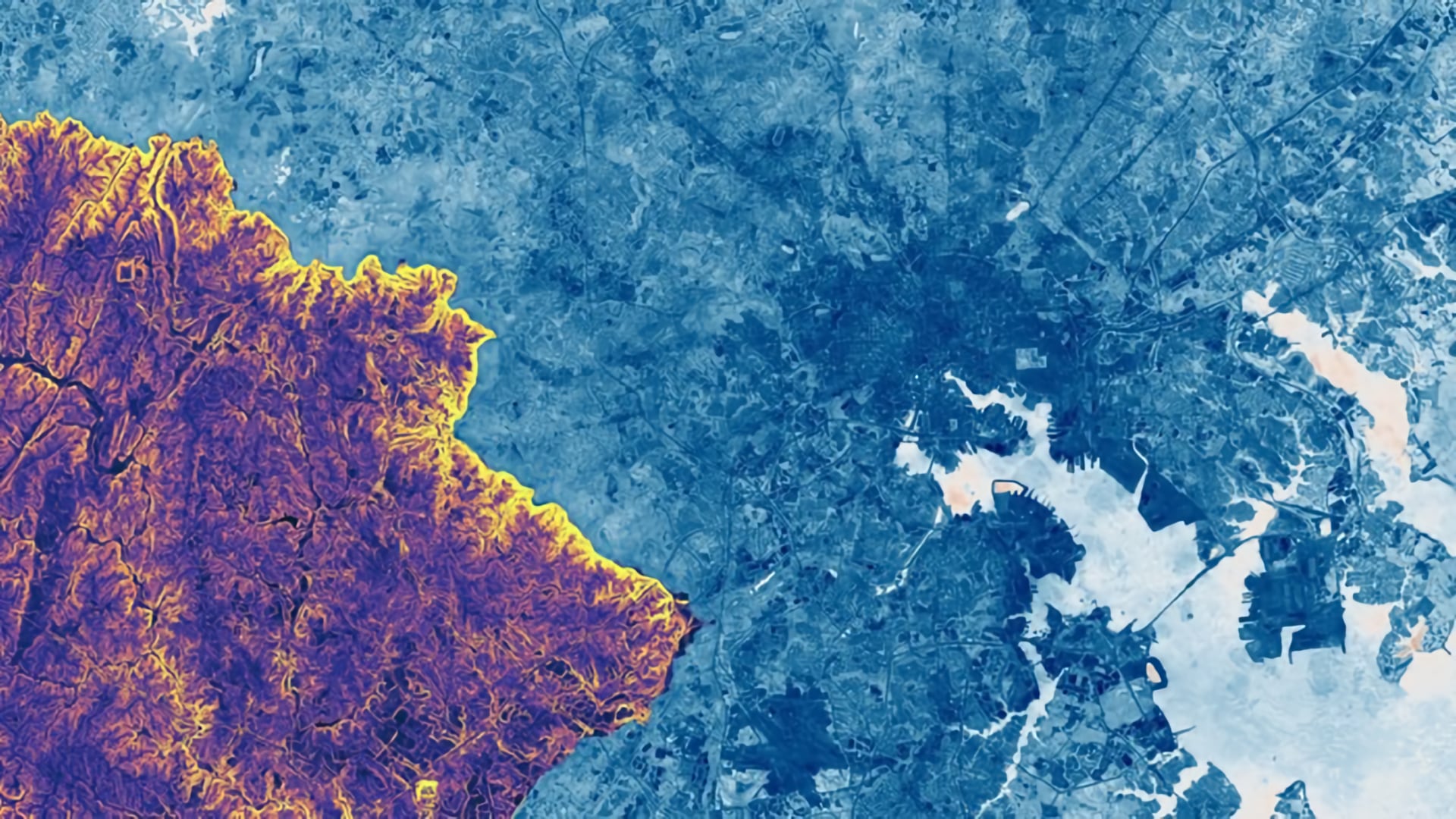
As flooding events in the United States grow in frequency and intensity, the use of technological advancements and applied science are increasingly necessary for effective flood monitoring and warning systems. The NASA DEVELOP Ellicott City Disasters II project investigated the use of machine learning for applications in flood risk detection to support the improvement of early warning systems. To strengthen the efforts of the Howard County Office of Emergency Management (OEM) in building a more robust flood monitoring system, the project improved the original statistical flood risk model, FLuME (Flood Learning Model Environment), programmed by the first DEVELOP term The enhancements incorporated an additional six years of precipitation and soil moisture data from the North American Land Data Assimilation System (NLDAS), modeled using Aqua Advanced Microwave Scanning Radiometer for EOS and Tropical Rainfall Measuring Mission TRMM Microwave Imager. These Earth observations were supplemented by stream gauge data from the OEM and the US Geological Survey. The resultant flood risk model FLASH (Flood Learning Environment and Severity Assessment Hub) was trained to evaluate input variables and predict stage height in Ellicott City in real time. The addition of an advanced deep learning framework known as long short-term memory improved the model’s ability to capture relationships between variables. To assess the effectiveness of the new model, FLASH produced a model efficiency metric of 0.99, a significant improvement over the 0.85 value produced by the previous model. The project assisted the OEM in pursuing the integration of open data and NASA Earth observations into a threat matrix capable of informing near real-time decision making.