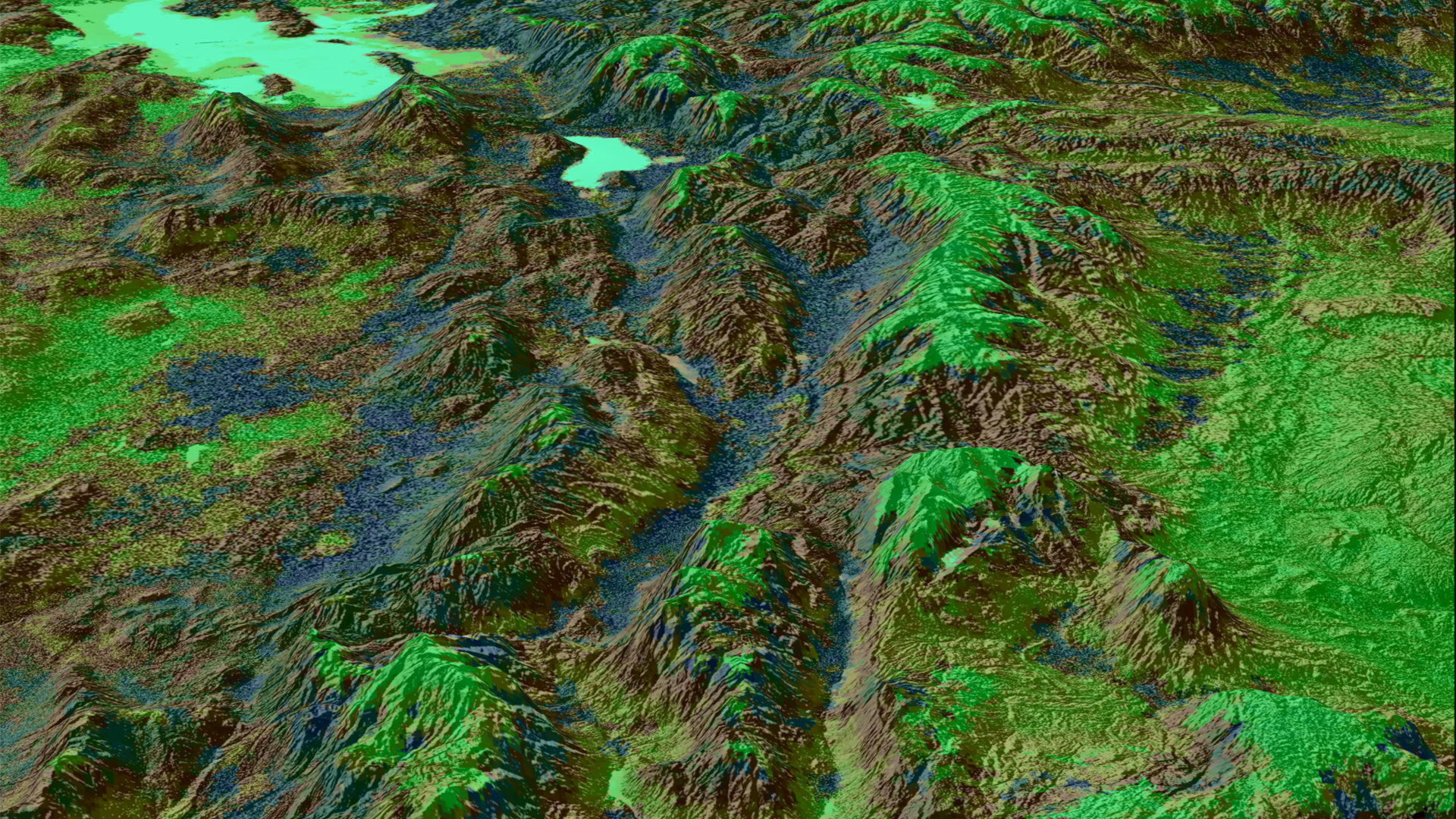
The eastern Great Basin (EGB) covers approximately 411,000 km2 within the states of Arizona, Colorado, Idaho, Utah, and Wyoming. Since the 1950s, wildfires have increased in both frequency and size within the EGB and neighboring states. Partners at the Bureau of Land Management (BLM), the Idaho Department of Fish and Game, the National Weather Service, and the Great Basin Coordination Center (GBCC) are particularly concerned with Live Fuel Moisture (LFM). Living vegetation that fuels wildfires, referred to as live fuel, requires greater energy input to combust when wet and less energy input to combust when dry, making LFM a vital measurement for predicting wildfire risk and severity. To increase spatial coverage for the EGB from the 155 in situ observation sites, the NASA DEVELOP team modeled LFM using satellite data from Aqua and Terra Moderate Resolution Imaging Spectroradiometer (MODIS) and Suomi National Polar-orbiting Partnership (NPP) Visible Infrared Imaging Radiometer Suite (VIIRS). The team incorporated remotely sensed data into machine learning modeling techniques, such as the Random Trees Classifier through ArcGIS Pro, to develop a predictive model of LFM. The remotely sensed data included vegetation indices, land surface temperature, evapotranspiration, and topographic variables. Model accuracy was evaluated by testing generated values against historical data obtained from partners at the BLM and the GBCC. The LFM model benefitted partners by improving the spatiotemporal resolution for wildfire forecasts. While model accuracy averaged at 8.2%, the LFM trend developed from model classification was useful for resource allocation and improved emergency response to wildfires within the EGB.