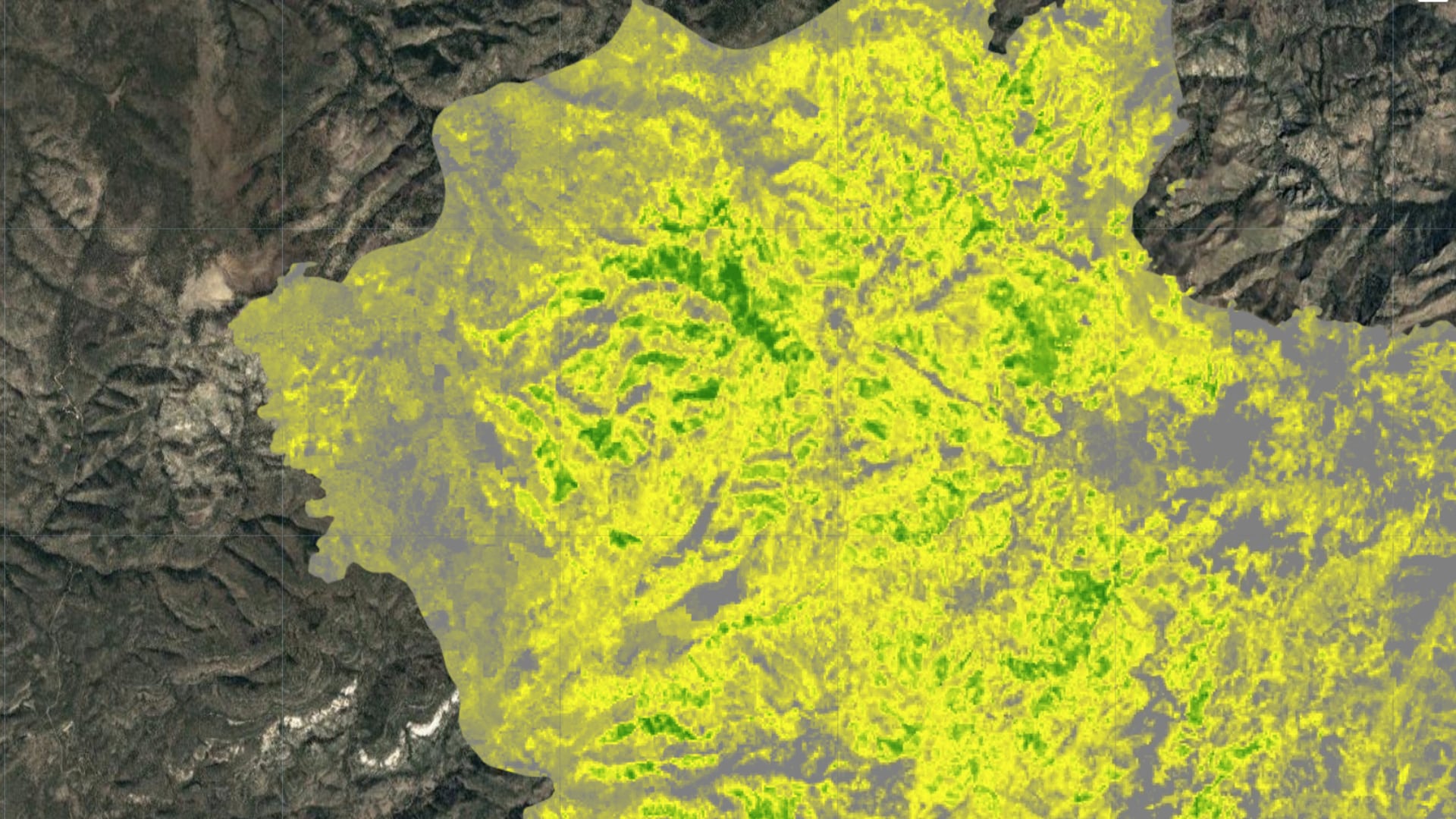
In recent years, wildfires in New Mexico’s Gila National Forest have become increasingly common and more severe. Wildfires can have powerful impacts on hydrology and soil stability, including erosion, flooding, and debris-flows that threaten lives and infrastructure downstream. Vegetation restoration treatments like seeding and mulching can mitigate these effects and facilitate ecosystem recovery. Understanding the effectiveness of various restoration methods is vital to planning a cost-effective and successful post-fire recovery strategy. The immediate response to a fire on US Forest Service land is coordinated by a Burned Area Emergency Response (BAER) team, a group responsible for mitigating immediate post-fire risks to human life, property, and critical natural and cultural resources. This study created a proof-of-concept methodology for a decision-support tool designed to help BAER teams identify the restoration treatments most likely to succeed in a given burned area. Leveraging random forest regression, Google Earth Engine, and Landsat 7 and 8 Earth observations, this study modeled vegetation recovery after the 2013 Silver Fire for seeded areas, seeded/mulched areas, and untreated areas. Treatment type and initial burn severity were the largest drivers of vegetation recovery across the landscape. Seeded/mulched areas showed higher recovery levels than untreated areas three months post-fire, but by four years post-fire, treated and untreated areas displayed similar recovery levels. To produce a robust predictive tool for the Gila National Forest, the model should be trained on many more fires and incorporate post-fire weather conditions into the process. Such a model will help partners ensure efficient resource use and plan effective post-fire restoration strategies.